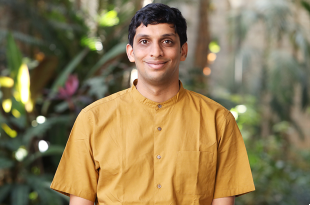
ABSTRACT: This paper presents a novel importance sampling (IS) scheme for estimating distribution tails of performance measures modeled with a rich set of tools, such as linear programs, integer linear programs, piecewise linear/quadratic objectives, feature maps specified with deep neural networks, etc. The conventional approach of explicitly identifying efficient changes of measure suffers from feasibility and scalability concerns beyond highly stylized models because of their need to be tailored intricately to the objective and the underlying probability distribution. This bottleneck is overcome in the proposed scheme with an elementary transformation that is capable of implicitly inducing an effective IS distribution in a variety of models by replicating the concentration properties observed in less rare samples. This novel approach is guided by developing a large deviations principle that brings out the phenomenon of self-similarity of optimal IS distributions. The proposed sampler is the first to attain asymptotically optimal variance reduction across a spectrum of multivariate distributions despite being oblivious to the specifics of the underlying model. Its applicability is illustrated with contextual shortest-path and portfolio credit risk models informed by neural networks.
Authors’ Names: Anand Deo, Karthyek Murthy
Journal Name: Operations Research
URL: https://pubsonline.informs.org/doi/10.1287/opre.2021.0331
Journal Article: 'Achieving Efficiency in Black-Box Simulation of Distribution Tails with Self-Structuring Importance Samplers' Prof. Anand Deo
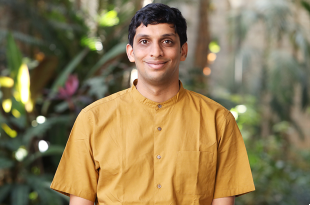
ABSTRACT: This paper presents a novel importance sampling (IS) scheme for estimating distribution tails of performance measures modeled with a rich set of tools, such as linear programs, integer linear programs, piecewise linear/quadratic objectives, feature maps specified with deep neural networks, etc. The conventional approach of explicitly identifying efficient changes of measure suffers from feasibility and scalability concerns beyond highly stylized models because of their need to be tailored intricately to the objective and the underlying probability distribution. This bottleneck is overcome in the proposed scheme with an elementary transformation that is capable of implicitly inducing an effective IS distribution in a variety of models by replicating the concentration properties observed in less rare samples. This novel approach is guided by developing a large deviations principle that brings out the phenomenon of self-similarity of optimal IS distributions. The proposed sampler is the first to attain asymptotically optimal variance reduction across a spectrum of multivariate distributions despite being oblivious to the specifics of the underlying model. Its applicability is illustrated with contextual shortest-path and portfolio credit risk models informed by neural networks.
Authors’ Names: Anand Deo, Karthyek Murthy
Journal Name: Operations Research
URL: https://pubsonline.informs.org/doi/10.1287/opre.2021.0331